AI Palette: Serving Up the Future of Flavours with AI
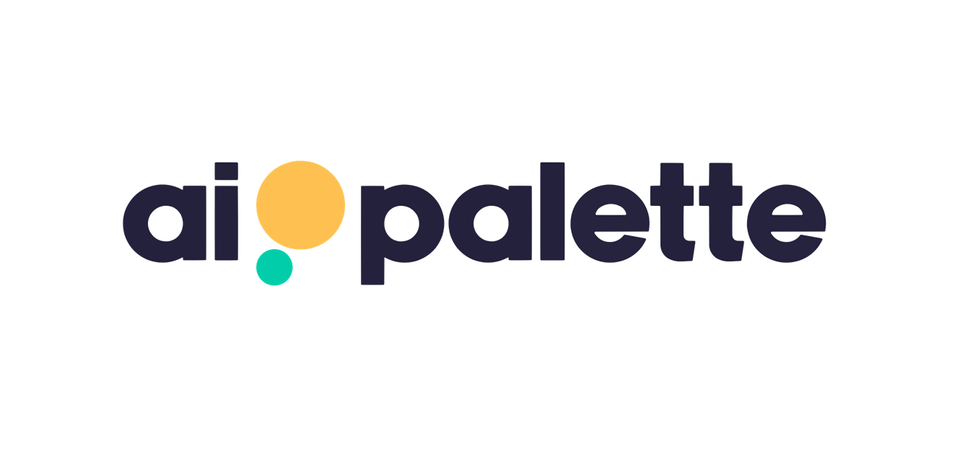
Somsubhra GanChoudhuri is the co-founder and CEO of AI Palette, an AI-driven platform that helps food and beverage companies predict emerging product trends. In this edition of Thought Leaders in Tech, we explore Somsubhra's startup journey, his advice for founders, and a deep dive into the tech behind AI Palette’s innovative solution.
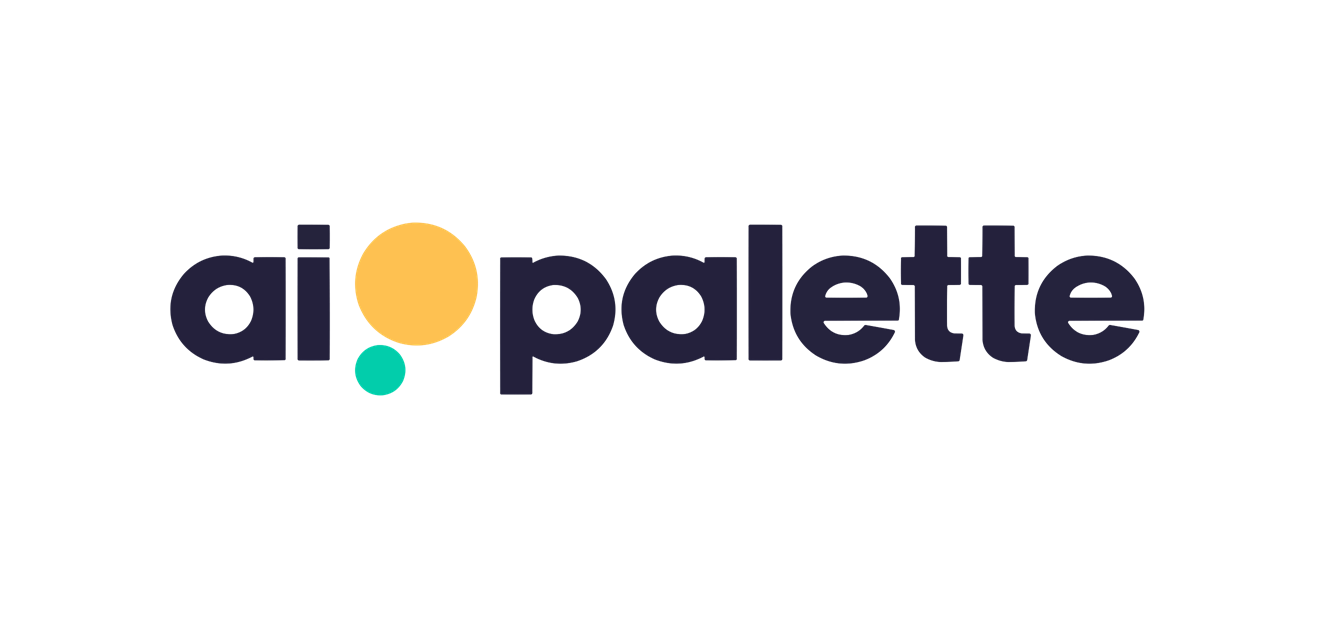
Co-founders: 2 (Somsubhra GanChoudhuri, Himanshu Upreti)
Employees: 100+
Amount Raised: $12.7M USD
Core Technology: AI-powered predictive analytics for the CPG (Consumer Packaged Goods) industry
Key Milestones:
- Signed first paying customer in the first year
- 65+ global brands, including Cargill, Diageo, Ajinomoto, Symrise, Mondelez, and L’Oréal, use AI Palette
- Every new product launched has secured a paying client within months
- Expanded into Beauty & Personal Care (BPC), onboarding one of India’s largest BPC companies within weeks
- Launched multiple new product lines in the last two years, creating a unified suite for brand innovation
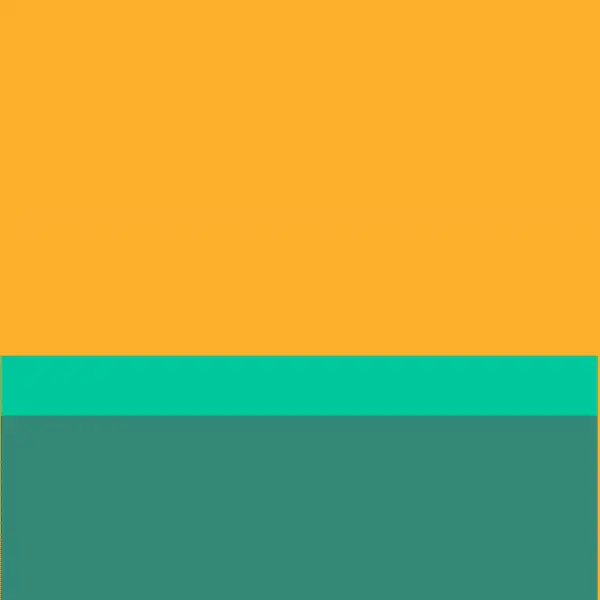
The Story
Following in his great-grandfather's footsteps to pioneer an industry
Early Inspiration
I’m Somsubhra–"Som" for short—the co-founder and CEO of AI Palette. Growing up, I was deeply inspired by the story of my great-grandfather in India. In the 1950s, just after India gained independence, the region where I come from had no school. Recognising the need for education, he decided to build one—a simple mud house where children could learn. After the first year, however, a flood came and destroyed it. He didn’t give up. He realised mud wasn’t strong enough, so the next time, he built it with bamboo. After the second year, some anti-socials came and burned it down. But they could not burn his ambition or his resolve. He refused to give in and, for the third time, he built the school—this time using concrete. To this very day, that school still stands and has become one of the best in the state, and his legacy is remembered by a road named after him.
That story shaped my mindset from a young age. It taught me that if you truly want to achieve something meaningful, setbacks are just part of the journey—you have to keep going no matter what. As I grew older, I started thinking about the best way to create a lasting impact in the world. I realised that one of the most powerful ways to do so is by building something that has never existed before. When you create something new, you bring innovation into the world. But beyond that, I saw how building a company could create jobs, drive economic growth, and bring in foreign investment. That’s when I knew—entrepreneurship was the path that would allow me to make the biggest difference. The lessons of perseverance and resilience that I learnt from my great-grandfather are the same principles that drive me today as an entrepreneur.
Founding AI Palette
Before starting AI Palette, I worked at Givaudan, the world’s largest flavour and fragrance company, supplying ingredients to major consumer packaged goods (CPG) brands. If you walk into a supermarket, chances are that one in three products you see contains either Givaudan’s flavours or fragrances.
During my time there, I worked closely with some of the biggest names in the industry—Nestlé, Unilever, and regional giants like Karuta Foods—down to smaller, emerging brands. Across the board, I noticed they all faced similar challenges when it came to understanding and predicting consumer trends. In my last role at Givaudan, I was part of the global strategy team, tasked with identifying future opportunities and the technologies worth investing in. That’s when I first encountered AI and machine learning.
It quickly became clear to me that AI was going to transform the CPG industry—it was just a matter of time. My first instinct was to find an AI/ML solution provider to onboard for Givaudan. But that was in 2018, long before AI had gone mainstream, so I found nothing tailored to the industry. That left me with two choices: wait for someone else to build it, or create it myself. I chose the latter. I quit my corporate job, partnered with my co-founder, Himanshu Upreti, and started AI Palette.
Today, AI Palette has grown into a 100-person company with customers across five continents. Most of them are Fortune 500 brands, proving that even the biggest players needed the kind of AI-powered insights we provide. We’ve secured a patent, filed five trade secrets, and, most importantly, pioneered one of the first AI solutions built specifically for the CPG industry. Looking back, I’m proud that we didn’t just predict the future of CPG—we helped shape it.
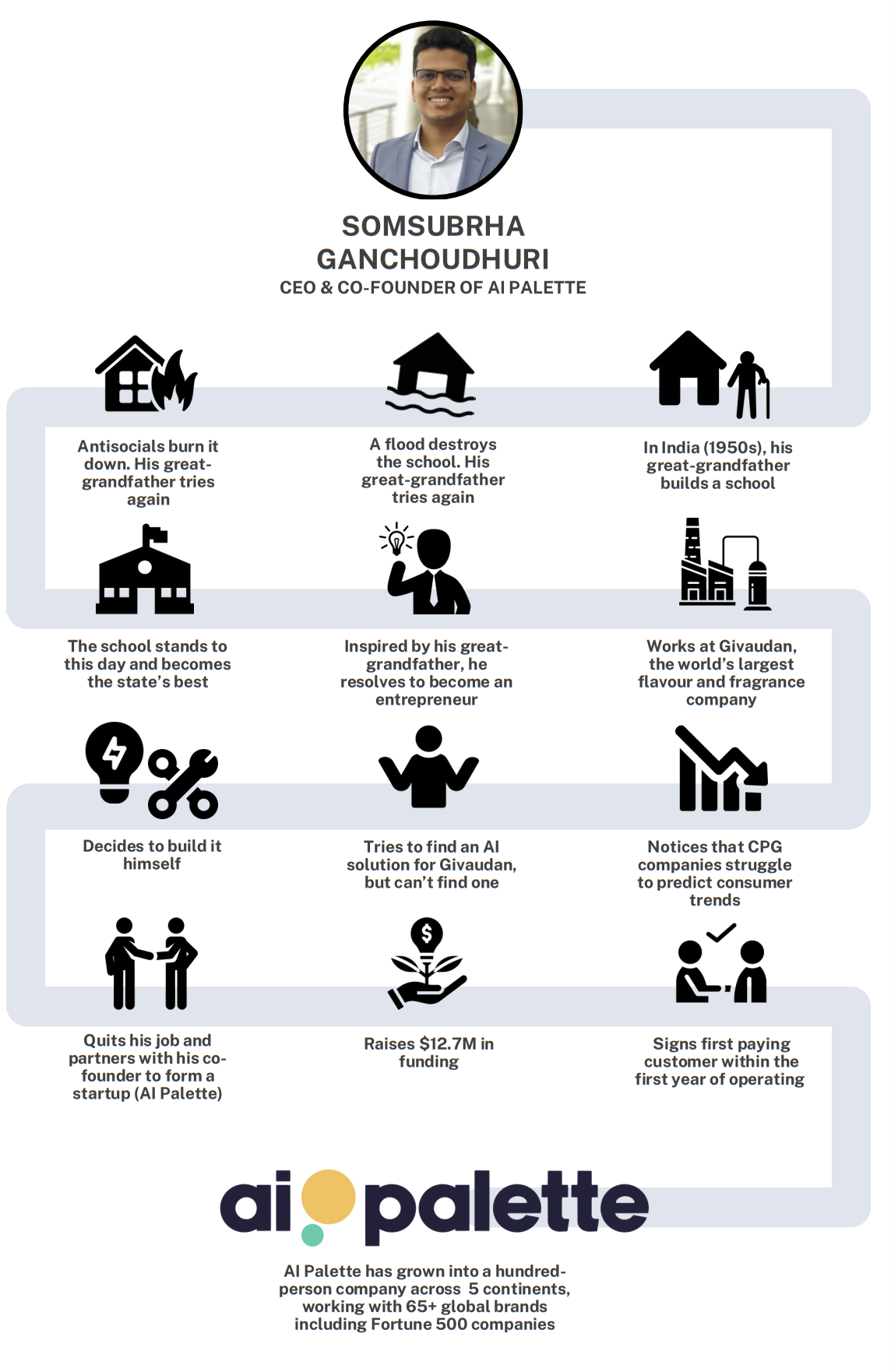
The Startup
Delivering a tasteful experience to the CPG industry
Ideation & Validation
Identify the pain points in your industry for ideas
When I was working in the flavour and fragrance industry, I noticed a major issue CPG companies faced: launching a product took at least one to two years. For instance, if a company decided today to launch a new juice, it wouldn’t hit the market until 2027. This long timeline made it difficult to stay relevant and on top of trends. Another big problem I noticed was that companies relied heavily on market research to determine what products to launch. While this might work for current consumer preferences, it was highly inefficient since the product wouldn’t actually reach the market for several years. By the time the product launched, the consumer trends had already shifted, making that research outdated.
That’s where AI can play a crucial role. Instead of looking at what consumers like today, we realised that companies should use AI to predict what they will want next. This allows businesses to create products that are ahead of the curve. Right now, the failure rate for new product launches is alarmingly high, with 8 out of 10 products failing. By leveraging AI, companies can avoid wasting resources on products that won’t succeed, leading to better, more successful launches.
Start by talking to as many industry experts as possible to identify the real problems
When we first had the idea for AI Palette, it was just a hunch, a gut feeling—we had no idea whether people would actually pay for it. To validate the idea, we reached out to as many people as we could within the industry. Since our focus area was all about consumer insights, we spoke to professionals in the CPG sector, particularly those in the insights departments of CPG companies. Through these early conversations, we began to see a common pattern emerge and identified the exact problem we wanted to solve.
Don’t tell people what you’re building—listen to their frustrations and challenges first.
Going into these early customer conversations, our goal was to listen and understand their challenges without telling them what we were trying to build. This is crucial as it ensures that you can gather as much data about the problem to truly understand it and that you aren't biasing their answers by showing your solution.
This process helped us in two key ways:
- First, it validated that there was a real problem in the industry through the number of people who spoke about experiencing the same problem.
- Second, it allowed us to understand the exact scale and depth of the problem—e.g., how much money companies were spending on consumer research, what kind of tools they were currently using, etc.
Narrow down your focus to a small, actionable area to solve initially.
Once we were certain that there was a clear problem worth solving, we didn’t try to tackle everything at once. As a small team of two people, we started by focusing on a specific area of the problem—something big enough to matter but small enough for us to handle. Then, we approached customers with a potential solution and asked them for feedback. We learnt that our solution seemed promising, but we wanted to validate it further.
If customers are willing to pay you for the solution, it’s a strong validation signal for market demand.
One of our early customer interviewees even asked us to deliver the solution, which we did manually at first. We used machine learning models to analyse the data and presented the results in a slide deck. They paid us for the work, which was a critical moment. It meant we had something with real potential, and we had customers willing to pay us before we had even built the full product. This was the key validation that we needed.
By the time we were ready to build the product, we had already gathered crucial insights from our early customers. We understood the specific information they wanted and how they wanted the results to be presented. This input was invaluable in shaping the development of our final product.
Building & Product Development
Start with a simple concept/design to validate with customers before building
When we realised the problem and solution, we began by designing the product, but not by jumping straight into coding. Instead, we created wireframes and user interfaces using tools like InVision and Figma. This allowed us to visually represent the product without the need for backend or frontend development at first. The goal was to showcase how the product would look and feel, helping potential customers understand its value before we even started building.
We showed these designs to potential customers and asked for feedback. Would they want to buy this product? Would they pay for it? We didn’t dive into actual development until we found a customer willing to pay a significant amount for the solution. This approach helped us ensure we were on the right track and didn’t waste time or resources building something customers didn’t actually want.
Deliver your solution using a manual consulting approach before developing an automated product
Initially, we solved problems for customers in a more "consulting" manner, delivering insights manually. Recall how I mentioned that when one of our early customer interviewees asked us to deliver the solution, we initially did it manually by using machine learning models to analyse the data and presenting the results to them in a slide deck. This works for the initial stages of validating your solution, as you don't want to invest too much time into building a full-blown MVP before understanding the exact features and functionalities that your users want.
However, after confirming that customers were willing to pay for what we provided, we moved forward with actual product development. This shift from a manual service to product development was key to scaling in a sustainable manner, as our building was guided by real-world feedback and insights rather than intuition.
Let ongoing customer feedback drive iteration and the product roadmap
Once we built the first version of the product, it was basic, solving only one problem. But as we worked closely with customers, they requested additional features and functionalities to make it more useful. As a result, we continued to evolve the product to handle more complex use cases, gradually developing new modules based on customer feedback.
Product development is a continuous process. Our early customers pushed us to expand features and modules, from solving just 20% of their problems to tackling 50–60% of their needs. These demands shaped our product roadmap and guided the development of new features, ultimately resulting in a more complete solution.
Revenue and user numbers are key metrics for assessing product-market fit. However, critical mass varies across industries
Product-market fit (PMF) can often be gauged by looking at the size of your revenue and the number of customers you're serving. Once you've reached a certain critical mass of customers, you can usually tell that you're starting to hit product-market fit. However, this critical mass varies by industry and the type of customers you're targeting. For example, if you're building an app for a broad consumer market, you may need thousands of users. But for enterprise software, product-market fit may be reached with just a few dozen key customers.
Compare customer engagement and retention with other available solutions on the market for product-market fit
Revenue and the number of customers alone isn't always enough to determine if you're reaching product-market fit. The type of customer and the use case for your product also matter. The level of engagement with your product—how much time users are spending on the platform—is also an important metric to track. The more time they spend, the more likely it is that your product is meeting a crucial need.
Another way to evaluate product-market fit is by assessing retention, i.e whether users are returning to your platform and relying on it consistently, as compared to other solutions available. That's another key indication that your solution is gaining traction in the market.
Business Model & Monetisation
Prioritise scalability
Initially, we started with a consulting-type model where we tailor-made specific solutions for each customer use-case we encountered and delivered the CPG insights manually, but we soon realized that this wasn't scalable. The problem with consulting is that you need to do the same work repeatedly for every new project, which requires a large team to handle the workload. That is not how you sustain a high-growth startup. To solve this, we focused on building a product that would address the most common problems faced by our customers. Once built, this product could be sold to thousands of customers without significant overheads, making the business scalable.
With this in mind, we decided on a SaaS (Software as a Service) business model. The benefit of SaaS is that once you create the software, you can sell it to many customers without adding extra overhead. This results in a business with higher margins, where the same product can serve many customers simultaneously, making it much more efficient than the consulting model.
Adopt a predictable, simplistic business model for efficiency. Look to industry practices for guidance
When it came to monetisation, we considered the needs of our CPG customers, who I knew from experience were already accustomed to paying annual subscriptions for sales databases and other software services. We decided to adopt the same model and charge our customers an annual upfront fee. This model worked well for our target market, aligning with industry standards and ensuring stable, recurring revenue. Moreover, our target CPG customers were already used to this business model and didn't have to choose from a huge variety of payment options, making closing sales a straightforward and efficient process.
Marketing & Sales
Educate the market to position yourself as a thought leader
When we started, AI was not widely understood, especially in the CPG industry. We had to create awareness around both AI and its potential value. Our strategy focused on educating potential users and customers about AI, its relevance, and why they should invest in it. This education was crucial to the success of our marketing efforts.
To establish credibility, we adopted a thought leadership approach. We wrote blogs on the importance of AI and how it could solve problems for CPG companies. We also participated in events and conferences to demonstrate our expertise in applying AI to the industry. This helped us build our brand and reputation as leaders in the AI space for CPG, and word-of-mouth spread as customers recognized us as the go-to company for AI solutions.


Leverage content marketing and online presence to attract media coverage
Online content, especially on LinkedIn, became a key marketing channel for us. Since most of our customers were enterprise-focused, LinkedIn was the ideal platform. The content we published not only helped educate the market but also led to significant media coverage. Publications in the food, beverage, and personal care industries began sharing our insights, broadening our reach and boosting our visibility. They would ask us for our thoughts and insights, quote us in their own content which they would then publish to their audiences, giving us a wider reach within the CPG industry and increasing our overall brand presence.

CNBC's feature on AI Palette in 2024
For B2B startups targeting enterprises, use LinkedIn
Personally, as the founder of a B2B enterprise technology company, I find that LinkedIn is the best social media channel for us. I think about 90% of our users will be on LinkedIn given the platform's more corporate-centric professional appeal, so it's become our primary content marketing channel.
Use events and conferences for direct exposure
Events and conferences also played an important role in marketing our business. Attending and speaking at these events allowed us to directly engage with potential customers and demonstrate the value of AI in solving their challenges. This helped us establish an authoritative presence in the CPG market and contributed to long-term brand building.
Start with founder-led, direct sales
In the early stages, founder-led sales are essential. As the founder, you're the best person to sell your product because you have the most intimate knowledge of it. Additionally, when you're just starting out, you can't afford to hire a sales team yet, so you you have to take on the responsibility of selling directly to customers. This approach also gives you valuable direct feedback that can be used to refine your product.
For the first year or two, I handled all of the sales for AI Palette. This involved talking to people in marketing and consumer insights within client organizations, demonstrating how our AI product could solve their problems, running product demos, and starting with pilot projects. Once the pilot produced positive results, we would scale the product across the organization.
Always charge something, even for pilots/POCs
When selling large enterprise products, the stakes are high, and customers are making significant financial commitments. Unlike consumer software, which may only be around fifty to hundrers of dollars, B2B products tend to be in the high thousands. Very quickly, contracts for these kinds of products can go from five figures to six figures, so most enterprise customers will want to do a pilot or POC before making any significant commitment. However, it's crucial not to offer free pilots, as it can be hard to gauge whether a customer is serious about the product. Charging—even a small amount—ensures that they are committed to using the product properly.
It’s tempting for startups to offer products for free in the hopes of gaining early traction with customers, but this approach doesn't work in the long run. Free offerings don’t establish the value of your product, and customers may not take them seriously. You should always charge for pilots, even if the fee is minimal, to ensure that the customer is serious about potentially working with you, and that they are committed and engaged with the product.
Pilots/POCs/Demos should aim to give a "flavour" of what you can deliver
A paid pilot/POC trial also gives you the opportunity to provide a “flavour” of what your product can deliver, helping to build confidence and trust with the client. It allows customers to experience a detailed preview of what your product can do, which builds anticipation and desire for the full functionality. During this phase, ensure your product is built to give them a taste of the value you can provide, which sets the stage for a broader, more impactful adoption down the line.

Fundraising & Financial Management
Leverage PR to generate inbound interest from VCs
When it comes to fundraising, our approach was fairly traditional—we reached out to VCs and used connections from existing investors to make introductions. However, looking back, one thing that really helped us build momentum during our fundraising process was getting featured in Tech in Asia. This wasn’t planned; it just so happened that Tech in Asia was doing a series on AI startups in Southeast Asia and they reached out to us for an article. During the interview, they asked if we were fundraising, and we mentioned that we were. As a result, several VCs we hadn’t yet contacted reached out to us. This inbound interest was incredibly valuable, and we found it far more effective than our outbound efforts. So, if you can, try to generate some PR attention—it can help create inbound interest from VCs, and that interest is typically much stronger and more promising than any outbound strategies because they've gone out of their way to reach out to you.
Be well-prepared and deliberate about fundraising. Keep trying and don't lose heart
When pitching to VCs, it’s crucial to be thoroughly prepared, as you typically only get one shot at making an impression. If you mess up, it’s unlikely they’ll give you a second chance. You need to have key metrics at your fingertips, especially if you're running a SaaS company. Be ready to answer questions like: What’s your retention rate? What are your projections for the year? How much will you close? What’s your average contract value? These numbers should be at the top of your mind.
Additionally, fundraising should be treated as a structured process, not something you do on the side while juggling other tasks. When you start, create a clear plan: identify 20 VCs to reach out to each week. By planning ahead, you’ll maintain momentum and speed up the process. Fundraising can be exhausting and disheartening, especially when you face multiple rejections. Remember, you just need one investor to say yes to make it all worthwhile.

When using funds, prioritise profitability and grow only when necessary. Don't rely on funding to survive.
In the past, the common advice for startups was to raise money, burn through it quickly, and use it to boost revenue numbers, even if that meant operating at a loss. The idea was that profitability wasn’t the main focus, and the goal was to show rapid growth for the next funding round. However, times have changed, especially with the shift from “funding summer” to “funding winter.”
My advice now is to aim for profitability as soon as possible and grow only when it's truly needed. For example, it’s tempting to hire a large team when you have substantial funds in the bank, but ask yourself: Do you really need 10 new hires, or could you get by with just four? Growing too quickly can lead to unnecessary expenses, so focus on reaching profitability as soon as possible, rather than just inflating your team or burn rate.
The key takeaway is to spend your funds wisely and only when absolutely necessary to reach profitability. You want to avoid becoming dependent on future VC investments to keep your company afloat. Instead, prioritize reaching break-even as quickly as you can, so you're not reliant on external funding to survive in the long run.
Team-Building & Leadership
Look for complementary skill sets in co-founders
When choosing a co-founder, it’s important to find someone with a complementary skill set, not just someone you’re close to. For example, I come from a business and commercial background, so I needed someone with technical expertise. That’s when I found my co-founder, Himanshu, who had experience in machine learning and AI. He was a great match because his technical knowledge complemented my business skills, and together we formed a strong team.
It might seem natural to choose your best friend as your co-founder, but this can often lead to conflict. Chances are, you and your best friend share similar interests, skills, and backgrounds, which doesn’t bring diversity to the table. If both of you come from the same industry or have the same strengths, you may end up butting heads on how things should be done. Having diverse skill sets helps avoid this and fosters a more collaborative working relationship.
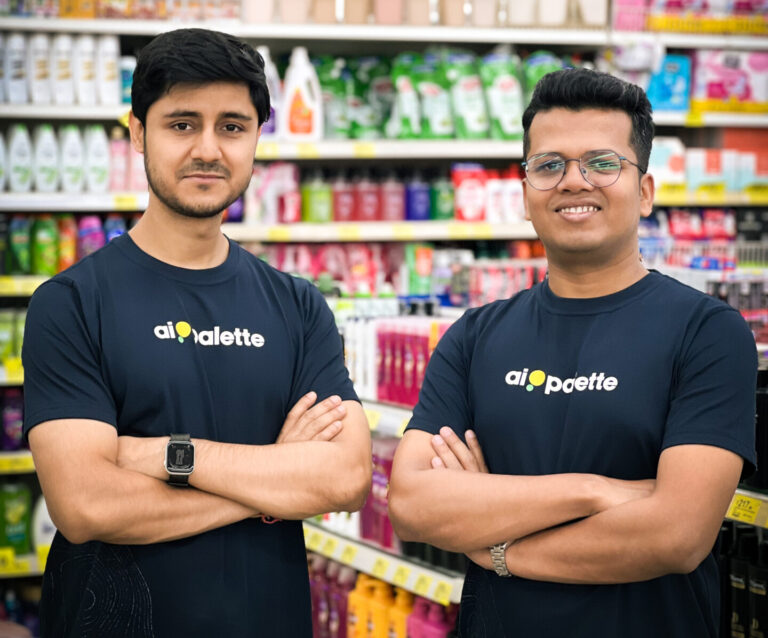
Define roles clearly to prevent co-founder conflict
To avoid conflict, it’s essential that your roles as co-founders are clearly defined from the beginning. If your co-founder and you have distinct responsibilities, there is no room for overlap or disagreement. This ensures that both of you can work without stepping on each other's toes, and there’s mutual respect for each other’s expertise.
This is another reason as to why it helps to have a co-founder with a complementary skillset to yours. Not only is having similar industry backgrounds and skillsets not particularly useful when building out your startup, it's also more likely to lead to conflicts since you both have similar subject expertise. On the other hand, if your co-founder is an expert in something that you're not, you're less likely to argue with them about their decisions regarding that aspect of the business and vice versa when it comes to your decisions.
Look for employees who are driven by your mission, not salary
For early-stage startups, the first hires are crucial. These employees need to be highly motivated and excited about the mission. Since the salary will likely be low and the work demanding, they must be driven by something beyond just the paycheck. The right employees are the swash-buckling pirates and romantics, i.e those who are genuinely passionate about the startup’s vision and want to be part of something impactful beyond material gains. When employees are motivated by the mission, they are more likely to stick around and help take the startup to greater heights.
A litmus test for hiring: Would you be excited to work with them on a Sunday?
One of the most important rounds in the hiring process is the culture fit round. This is where you assess whether a candidate shares the same values as you and your team. A key question to ask yourself is: "Would I be excited to work with this person on a Sunday?" If there’s any doubt about your answer, it’s likely not a good fit. The idea is that you want employees who align with the company's culture and values and who you would enjoy collaborating with even outside of regular work hours.
How we structure the team at AI Palette
We have three broad functions in our organization. The first two are the big ones:
- Technical Team – This is the core of our product and technology. This team is responsible for product development and incorporating customer feedback into improving the technology
- Commercial Team – This includes sales, marketing, customer service, account managers, and so on, handling everything related to business growth and customer relations.
- General and Administrative Team – This smaller team supports functions like finance, HR, and administration.
As with almost all businesses, we have teams that address the two core tasks of building (technical team) and selling (commercial team), but given the size we're at now, having the administraive team helps smoothen operations.

Set broad goals but let your teams decide on execution
What I've done is recruit highly skilled people who don't need me to micromanage them on a day-to-day basis. They're experts in their roles, and as Steve Jobs said, when you hire the right person, you don't have to tell them what to do—they understand the purpose and tell you what to do.
So, my job as the CEO is to set the broader goals for them, review the plans they have to achieve those goals, and periodically check in on progress. For example, if our broad goal is to meet a certain revenue target, I break it down across teams:
- For the sales team, I’ll look at how they plan to hit that target—how many customers they need to sell to, how many salespeople they need, and what tactis and strategies they plan to use.
- For the technical team, I’ll evaluate our product offerings—whether they think we need to build new products to attract more customers, and whether they think it's scalable for the number of customers we plan to serve.
This way, the entire organization's tasks are cascaded in alignment with our overarching goals, with me setting the direction and leaving the details of execution to the skilled team members that I hire.
The Tech
Leveraging AI to Predict Product Trends in Food and Beverage
The Problem: Understanding consumer trends in food and beverage
The food and beverage industry struggles to predict consumer trends and determine which products will succeed, often relying on outdated market research or intuition. Companies face difficulty identifying emerging trends quickly enough to capitalize on them, as consumer preferences are influenced by various factors like cultural shifts and social media. In my experience in the CPG industry, the success rate of new product launches is extremely low, with around 8 out of 10 newly-launched products failing on the market. So the failure rate is high, despite tons of corporate funding going towards creating and launching these new offerings.
Moreover, the data needed to make informed decisions on new consumer food products is unstructured and fragmented across platforms like online menus, social media, Google search trends, and e-commerce, and is typically unstructured. Traditional methods of analysis, typically based on a brand's own consumer data, are slow and insufficient, leaving businesses vulnerable to missed opportunities or misjudged demand. AI-driven solutions are essential for quickly processing and interpreting this data to provide timely, accurate insights.
The Solution: AI-driven insights and prediction
At AI Palette, we assist food and beverage companies in deciding what products to launch next. For instance, if a beverage company wants to introduce a new juice in Thailand, our platform helps them identify which types of juices are most likely to be successful. To do this, we analyze various online data sources related to the food and beverage industry to detect emerging patterns and trends in consumer preferences.

This includes looking at online menus to identify popular juices, scanning social media to assess consumer interest, examining Google search volumes to see what people are searching for, and reviewing e-commerce platforms like Amazon to track product listings. Our goal is to find out which products are in demand and understand shifting trends, such as juices that have recently gained interest.
The data available online is often unstructured, meaning it comes in different shapes and forms, which makes it difficult to analyze. We tackle this challenge by first structuring the data. We then use natural language processing (NLP) algorithms to interpret the language used by consumers. Since Southeast Asia has many non-English languages, we've developed a Thai NLP system to understand how Thai people talk about food and beverage in their region.
By identifying current trends from this data, we can also predict future consumer demand. Our AI models combine data from multiple sources to detect which juices are trending and which ones might see a surge in popularity, even if they're not currently widely known.
As technology evolves, so does the way we deliver results. With the rise of generative AI, we're shifting from traditional, complex charts to more conversational formats. Instead of providing analytical results through charts, we now deliver insights in a more user-friendly, presentation-style format, which can be generated directly through conversational AI. Users can interact with a chatbot that runs multiple filters and gives them detailed, actionable insights in real time, transforming how companies interact with data and make decisions.
Member discussion