Betterdata: Engineering Data Privacy with Generative AI

Uzair Javaid, a Ph.D. with a passion for data privacy, co-founded Betterdata to tackle one of AI's most pressing challenges: protecting privacy while enabling innovation. In this edition of Thought Leaders in Tech, Uzair shares his journey, the inspiration behind Betterdata, and the lessons learnt in building a privacy-first AI company.
The Story
Swapping academia for entrepreneurship, hacking the Ethereum blockchain and championing startup culture in Asia
Who Are You? What's Your Story?
My name is Uzair Javaid. I'm the CEO and co-founder of Betterdata, a startup focused on generative AI and privacy engineering to enable large-scale synthetic data generation and help enterprises with their AI/ML, Analytics, and Augmentation needs.
Originally, I am from the north of Pakistan from a city called Peshawar. I completed my undergraduate degree there in electrical engineering, graduating as the valedictorian. However, I always felt that I should have pursued computer science because of my natural affinity for coding. I was one of the best programmers in my batch, and coding logic came to me effortlessly.
During my undergraduate studies, I was fortunate to be taught by three exceptional teachers (Sir Naveed Aman, Asif Iqbal and Muhammad Ehtesham). The first two had pursued higher education abroad, one in the United States and the other in Sweden, bringing a wealth of advanced knowledge to their teaching. The third was an English literature teacher who helped me understand and process my emotions and thoughts in ways I couldn’t before. Because of these influences from teachers, I was determined to study abroad for advanced studies after my bachelor’s degree.
I initially enrolled in a master’s program in telecommunications at an Italian polytechnic institute in Milan (Polimi), but dropped out after a month because I didn’t find it fulfilling (so I’m a dropout as well). Returning to Pakistan in mid-2016, I started from scratch, applying for graduate programs both locally and internationally. In 2017, I received a Ph.D. scholarship to NUS (National University of Singapore), achieving my long-term goal of pursuing a doctorate without needing a master’s degree.
I moved to Singapore in early 2017, and my Ph.D. focused on computer engineering and computer science, specialising in data security and privacy. By 2021, as I was wrapping up my thesis, my final project involved ethically hacking the Ethereum blockchain, which I did. Just as I had developed a strong affinity for coding during my bachelor’s, I discovered a similar passion for privacy engineering after my Ph.D.
My Ph.D. program required five publications for graduation, but I published 15—three times the requirement. This drive pushed me to challenge myself further. While completing my Ph.D., I simultaneously pursued three goals: finishing my thesis, applying for jobs, and exploring startup ideas. One of these explorations led me to apply for Entrepreneur First, where I met Kevin Yee, who later became my co-founder. Kevin’s expertise was in generative models, and mine was in privacy. Together, we created a platform at the intersection of AI and privacy, which became Betterdata with a thesis that better data leads to better AI.
Looking back, when I arrived in Singapore in 2017, I didn’t even know what entrepreneurship meant (and I still don't!). However, I thrive in uncertainty and enjoy challenging myself daily, which building a startup allows me to do. I feel that I am exactly where I’m meant to be, channeling my passion for privacy engineering into Betterdata and building the foundations of AI.
Personally, I have two key driving forces: internal and external. Internally, I am driven by a desire to challenge myself. While academia allows for deep exploration of topics with more time and creative freedom, the startup industry operates at a faster pace with tighter deadlines and higher accountability. Externally, as an Asian, I am motivated by the global dynamics of technological innovation. Much of the world’s technological contributions come from the West, with Asia primarily seen as a consumer rather than a contributor. I aim to challenge this status quo by proving that world-class technologies can emerge from Asia too.
It's been almost three years since we started, and we launched the first version of our product early last year. Last year, we saw good progress on the problem-solution front. Now, we’re working towards achieving product-market fit. I’d say we’re currently about 40% of the way there, with the goal of reaching 60–70% within the next year 2025. As of today, our biggest milestone has been securing a contract with the US Department of Homeland Security. Out of the four companies awarded the contract globally, we’re the only one based in Asia.
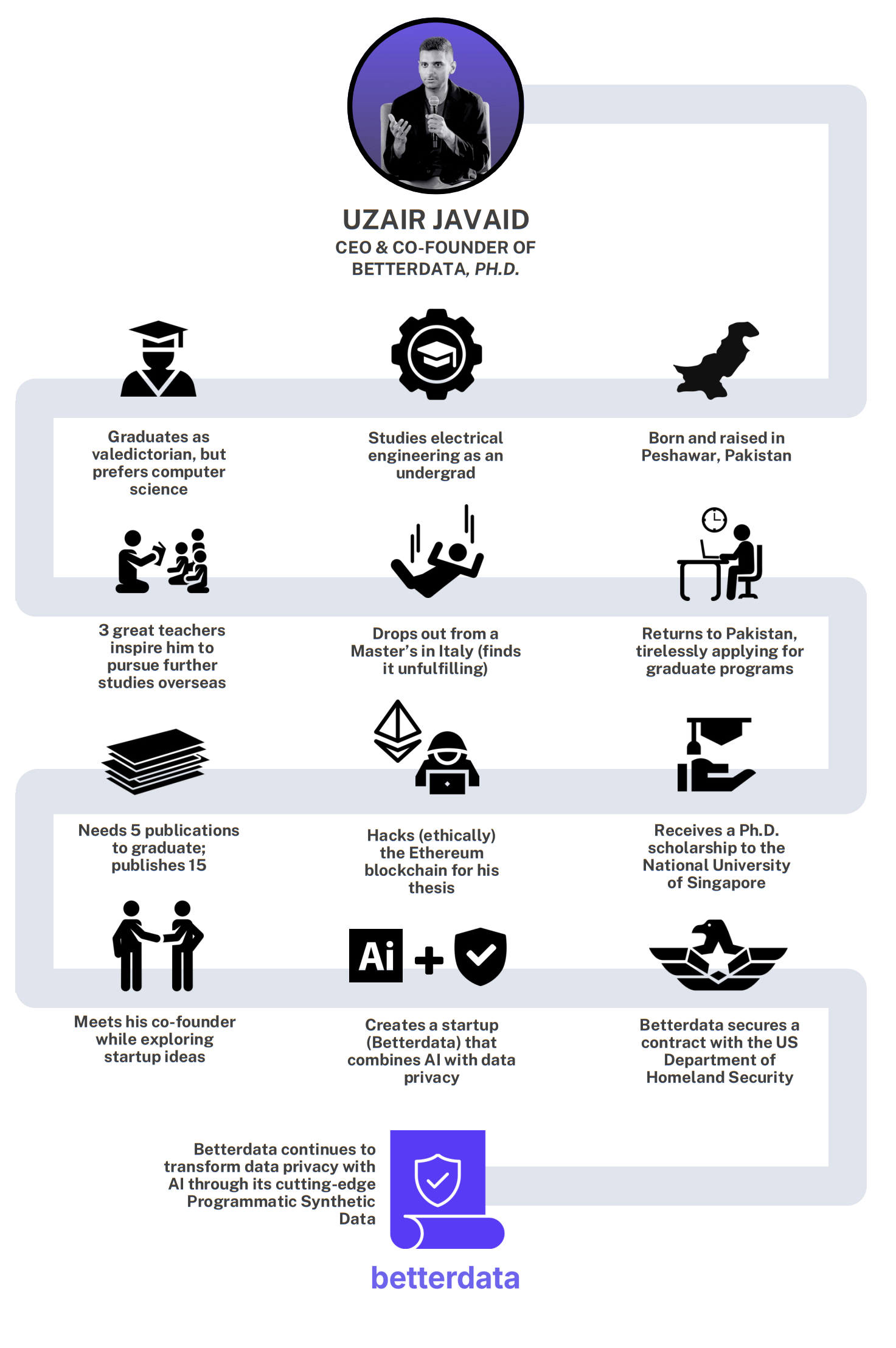
The Startup
Building a B2B AI + Data Privacy startup that’s used by the US Department of Homeland Security
(1) Ideation and Validation
Problem
The idea for me actually came from my Ph.D. My last project involved ethically hacking the Ethereum blockchain, where I managed to reverse-engineer how user wallets are created. In blockchain systems, instead of a password, you have a private key. I was able to reverse-engineer Ethereum's wallet-generation framework and gained access to 670 user wallets. This allowed me to access those wallets and take funds out without anyone even noticing (of course I never did that and reported my findings together with defensive measures in my Ph.D. thesis).
This experience made me realize that when we generalize the concept of using encryption for data sharing, there must be better alternatives. My affinity for privacy led me to explore this landscape. Broadly speaking, techniques in this domain can be divided into AI-based and non-AI-based methods. Back in 2021, I noticed that most approaches fell into the non-AI-based category. This prompted the thought: how can AI contribute here? That’s how we arrived at the idea of leveraging deep synthesis technology.
At that time, deepFakes were making waves, with people substituting faces with celebrities like Tom Cruise in humorous clips on YouTube. DeepFakes are essentially a kind of synthetic data generation that uses real data (e.g., celebrity face images) to generate synthetic media, such as altered videos or images, that appear to be real. Our idea was to use this same kind of technology, but instead apply it to tabular data to solve a real business problem.
Market Research and Validation
We conducted market research by identifying a significant opportunity stemming from the General Data Protection Regulation (GDPR) in Europe, which was enacted in May 2018. The GDPR affected over 28 million businesses globally, creating a huge market for privacy tech solutions. While this statistic validated the market size, it didn’t directly guide our product development.
The key for us was speaking to 50 potential customers to find convergence in their needs. These customers ranged from startups and SMEs to large MNCs. Through these discussions, we realized that companies with substantial private customer data, typically large MNCs or tier-one enterprises, were facing significant challenges around data access and data sharing.
These companies were not only willing to pay, but willing to pay a premium to comply with regulations and to leverage data for innovation. This customer feedback became a cornerstone for our development process. To stay aligned, we continue to engage with a healthy number of customers each month as a litmus test to ensure we remain on the right track.
Finding and Engaging Potential Customers
Personally, I dislike receiving cold calls, so I don’t believe in outbound strategies for enterprise sales. If I don’t believe in it, I can’t build a company that relies on it. Instead, we used three primary methods to engage potential customers for feedback and validation.
- Account-Based Marketing (ABM):Our users are technically savvy and mature, so we target them with content that directly addresses their specific problems. While many know about AI and synthetic data, it’s the niche problems that pique their interest. For instance, banks may require a platform capable of handling a billion customer transaction records. Open-source models often can’t scale to that extent. By presenting solutions tailored to these specific challenges, we attract interest.
- Targeted Content Marketing:Regularly speaking to customers informs us about their challenges, which in turn helps us create relevant content. This content is then used to target ideal customer profiles to reach out to, while also helping to establish ourselves as thought leaders in the privacy engineering and AI space.
- Raising Awareness Through Partnerships:There is a significant lack of awareness about privacy, especially in Asia, let alone using synthetic data to address privacy challenges. To tackle this, we collaborate with partners like Infocomm Media Development Authority in Singapore, the National University of Singapore, Singapore University of Technology and Design, and VC firm Plug and Play to share knowledge. We also contribute to advisory guidelines on data privacy with PDPC (Personal Data Protection Commission) Singapore, which helps build credibility and raise awareness for the problem and solution we are trying to validate.
The Magic Number: 50 Customer Conversations
The "magic number" of 50 isn’t arbitrary. Some suggest 100, but this is difficult for most founders starting out, so 50 strikes a practical balance. When speaking to customers, the goal is to find convergence. For example, out of 10 customers, if three mention the same problem or problem space, it indicates a pattern. By speaking to 50 customers, you’re likely to identify 10–15 customers with overlapping issues, which is a strong foundation on which to build a prototype.
The idea is to then build a prototype or MVP based on feedback from these conversations. If customers are willing to pay for it, that’s a strong signal of market demand. This approach ensures iterative improvement while remaining practical.
(2) Building and Product Development
Prototyping
Consult Your Customers Early On
In the early stages, we focused on building synthetic data generation algorithms and using those algorithms on open-source data sets for PoC (Proof-of-Concept). What we did was build synthetic data generation algorithms and a basic UI where users could upload test datasets, generate synthetic datasets, and then compare the two. To get started, we used five open-source datasets to generate results. We used these results to talk to banks and eventually secure PoCs and pilot programs. When we talked about our MVP, it was essentially a function of applying that approach to customer data. For example, if we wanted to work with a bank, the bank has real customer data, so whatever we offer has to work on their particular datasets.
By testing our early prototype with customers early on, we soon realised that banks would never let us work with their actual customer data outright because of privacy concerns. So we had this gap—on one side was the bank, and on the other, us, without any knowledge of how their real data looked. The goal was to bridge this gap with our MVP. Hence, when we conducted POCs, MVPs, and pilots, we would have to install our solution on-site and let the customer use it. They would then come back with feedback, telling us what worked and what didn’t. This feedback was consultative in nature since they couldn’t share their data directly. We then had to meet some of them in person or hop on calls to understand the scenarios they were facing.
Through this process of continuous consultation, we then stumbled upon another problem. While open-source data is clean and well-structured— you can see it, clean it, and manage it– real enterprise data is entirely different. Their schemas and structures are often not well-organised and pose unique challenges, like missing values. For instance, when we initially built a model using open-source datasets, those datasets didn’t have missing values. Missing values are common in real datasets—think of an Excel sheet where some cells are blank. When we did a POC with one customer, their data had missing values, and our early synthetic data generation pipeline broke down. That’s when we got the feedback to support missing values which helped us refine the pipeline accordingly. Missing values is one scenario and we have covered so many more since we started Betterdata.
It was this process of continuously consulting our customers and working closely with them that supercharged our product development efforts. By listening closely to your customers' feedback, as well as observing how they use your product and the problems they encounter while doing so, you can uncover valuable insights to improve your prototype, enhance UX, and address unmet needs. This iterative process not only strengthens your product but also fosters trust and loyalty with customers by demonstrating that you are responsive to their needs and committed to their success.
The Data Advantage
Even if we provide the best results today, whether it works on customer data is subjective to their private datasets that we have zero visibility on. The gap between what we know and what they know continues to evolve. As we move forward, the goal is to keep bridging this gap. Over time, this gives us more differentiation because if someone else comes up with the same idea as ours, they can’t claim the same insights or capabilities that we have. They wouldn’t know, for example, what a typical bank’s data looks like, which gives us a significant competitive edge. In other words, the more customers we tested on, the more our advantage grew because our algorithms were prepared for a wider variety of use-cases.
Iterating
When it comes to iteration and refining our product, my approach is simple: listen to your customers, and your customers only. It's crucial to block out the noise—whether it's external advice, trends, or hype—and focus entirely on what the customer needs since they're the only ones actually paying you. Here's how I think about it:
Focus on Feedback, Not External Noise
First and foremost, I emphasize that everyone on the team must be constantly asking one simple question: Which customer is asking for this feature or solution? As long as there’s a clear answer, we can move forward. The feedback we get directly from customers is our true guide. External influences or tech trends, while potentially helpful, often bring more noise than clarity. This is especially true when there's so much talk about "hot" technologies like AI. Differentiating between signal and noise is crucial. You might make a quick buck by jumping on a trend, but long-term success comes from solving real customer problems.
Focus on Problems, Not Exact Requests
While customers don’t always know exactly what they want, they are very aware of the problems they face. Our job is to identify those problems and find ways to solve them in a manner they have never seen before. This process often involves some back-and-forth where customers will often express a desire for certain features or capabilities, but we have to stay focused on solving the core problem rather than addressing their exact request since customers aren't usually in the best position to know how to solve their own problems. There's this quote often attributed to Henry Ford, where he allegedly said "If I asked people what they wanted, they would have said faster horses!". Whether or not Henry Ford actually said that, the basic sentiment holds true when eliciting customer feedback for product development.
Iteration Succeeds When Customers Keep Coming Back
We evaluate whether we’re on the right track by whether customers keep coming back to us. If they return with more feedback, more requests, or new problems, that’s a good sign that we’re addressing their pain points well or not-so-well (either way is fine). If they just say, “goodbye,” and walk away, we know we haven’t hit the mark. This ongoing feedback loop is what drives iteration.
I think the logic here is that when customers feel that you're helping meet their needs, they're more willing to keep working with you because it benefits them. On the other hand, if you're not doing a good job of improving the product based on their feedback, it is simply easier to part ways than to take the time and effort to guide you towards a better product. Your customers want to be helped with their problems; they do not want to have to do the helping.
Move Fast and Move On
In the early stages, it’s important to ship products that solve a problem, even if they’re not perfect. Speed matters. Our first version is about delivering something that solves the issue quickly. We then let customers decide whether it's good enough. If they say it’s good, we consider it a job well done. If they say it’s not, we go back to the drawing board and iterate.
However, not all customers are disciplined or clear in their feedback. As a company, we must maintain more discipline than our customers. If a customer isn’t giving useful feedback and is only wasting our time, we need to be willing to move on and focus our efforts on those who truly value our work. This is difficult, but necessary to avoid wasting valuable time and resources.
Focus On Your Niche
As we continue to evolve, it’s essential to stay focused on building our niche. Tech trends can be enticing, but we must remember that differentiation is what will sustain us in the long run. By staying true to the problem we're solving and being mindful of our customer needs, we can create something unique that stands out from the noise.
For our product, the core use case became generating synthetic data for privacy compliance. We decided to focus on the highly specific needs of industries like banking and government institutions, which deal with sensitive customer data and require secure, compliant systems. By zeroing in on this niche, we were able to build a solution that really catered to their pain points. This focus laid the foundation for our scaling strategy, which we are now using to expand beyond our initial markets and into the broader Asian market.
Product-Market Fit
When it comes to assessing product-market fit, we focus on two key metrics. These help us understand whether we are truly resonating with our target market and if we're headed in the right direction.
Metric 1– Customers' Willingness to Pay
The first metric we track is customers' willingness to pay. This is a straightforward way of gauging whether customers see enough value in what we're offering to part with their money. Here's how I approach this:
- Meeting Quantity: I keep track of how many meetings we can book each month, as well as the quality of those meetings. The more meetings we have, the more we know that there’s genuine interest in what we’re offering. We also pay close attention to the type of people we’re speaking with. Are they decision-makers or key stakeholders? This helps us validate whether we’re talking to the right audience.
- Meeting Quality: It's not just about the quantity of meetings, but also about the type of people we are speaking to. When we have meetings with people who are in positions to make purchasing decisions, that tells us we’re heading in the right direction.
At Betterdata, we applied this principle early on by focusing on getting meetings with the right people—mainly within banks and large enterprises. By tracking both the quantity and quality of these meetings, we were able to gauge whether our product resonated with our target audience. This feedback loop helped us refine our offering and better tailor our approach to meet the needs of potential customers.
Metric 2– How Much Customers are Willing to Pay
The second metric we look at is how much those paying customers are willing to pay. This is a bit more nuanced, as prices can fluctuate during the early stages, but ultimately, we need to identify our sweet spot. When customers are willing to pay a premium for what we're offering, it signals strong potential for product-market fit.
For us, we realized early on that large enterprises, especially those handling sensitive customer data (such as banks), were more willing (and able) to pay a premium for our synthetic data platform. We focused on targeting these companies because they had the budget and the need for privacy tech, especially in light of GDPR and ever-increasing data protection regulations globally. As we moved forward, we found that companies were not only willing to pay but were also willing to pay premium prices for solutions that helped them innovate using their data while maintaining compliance. This feedback from customers helped us refine our product further and build a roadmap to scale efficiently. In terms of scaling, I believe having a clear goal post and consistent execution is critical - it's not about working hard, but about working consistently towards defined objectives.
Once we had identified the top use cases where customers were both willing to pay and willing to pay higher ticket sizes, we focused on building our platform around these specific niches. This allowed us to ensure that we were meeting customer needs in the most impactful way.
By tracking customers willing to pay and focusing on the highest-value use cases, we’ve been able to refine our product and build a solid foundation for scaling.
(3) Business Model and Monetisation
This was an area I initially struggled with. I had a tendency to get overly educational in my sales pitches, rather than focusing on the core value proposition. We eventually developed a product roadmap with different models, allowing us to map customer requirements to specific offerings and justify our pricing accordingly. Our model includes project-based pilots as well as annual contracts for successful deployments.
Let The Customer Decide If They Need Your Product
When it came to deciding on Betterdata's business model and overall monetization strategy, I must admit, I was quite lost. As a CEO coming from an academic background, my early passion was primarily about educating people on the privacy space and the potential of synthetic data. I would often spend long hours explaining to anyone who would listen how synthetic data could solve most of their problems. So, if a customer told me they were using anonymization and didn't need synthetic data, I'd dive right into a 30-minute to 90-minute educational seminar (passion has no price right). The problem was that, despite all this education, we weren’t making any sales. We were just educating people, wasting a lot of time, and seeing no return on our efforts.
Over time, I learned a valuable lesson: sometimes, you need to let the customer decide if they have a problem that your solution can solve. Today, if a customer says they’re using anonymization and don’t need synthetic data, I simply tell them, “You don’t have any problem. You can solve your issues with what you have.” This shift in mindset helped us better qualify customers and avoid wasting time on those who weren’t ready to invest in our solution.
Categorise Your Offerings Based on Customers' Needs
As we began to refine how we would charge customers, it became clear that pricing had to align with the specific needs and questions of each customer. Early on, we spoke with a wide range of decision-makers—data scientists, Chief Data Officers, Chief Digital Officers—and they would ask all sorts of questions. Some were things we hadn’t even thought of or features we didn’t yet have in our platform. Initially, we tried to address every question and feature request, which created a lot of friction and confusion.
Eventually, after speaking with almost 100 customers, we created a product roadmap that categorized our offerings into different AI models. Each model had its own set of features. So, the next time we spoke to a customer, we would map their requirements to one, two, or all models, depending on their needs. This gave us a clearer way to justify pricing. For example, if a customer needed Model 1, they paid a specific price. If they needed Model 2 as well, the price would increase, and so on. This approach allowed us to manage customer expectations and prevent them from asking about features they didn’t need. If they wanted all the features, they would know they’d have to pay a higher price.
This also introduced focus into our customer conversations. We were able to clearly communicate that if they only needed one model, they didn’t have to ask about features from the other models. And if they were interested in the full suite of features, they would be expected to pay a premium for it (you see people get serious about what they want exactly when they know about pricing implications, otherwise it’s just window shopping). This structure helped us manage customer conversations more effectively, guiding them towards a more tailored solution that matched their requirements specifically.
Employ a Structured, Phase-Driven Approach
At Betterdata, our customer engagement process unfolds across three distinct phases, each with its own approach to pricing and payment.
Phase 1: Trial and Benchmarking
The first phase begins when we reach out to potential customers. During this stage, we aim to demonstrate the effectiveness of our solution, typically using open-source datasets to show benchmarks and results. This serves as a trial period for the customer, allowing them to evaluate the feasibility of our solution without committing to a full contract.
Phase 2: Pilot or PoC (Proof of Concept)
Once the customer finds the trial results attractive, we move into the second phase, which involves conducting a PoC or pilot with the customer’s private data only.
During the pilot, we work closely with the customer to refine the solution and ensure it fits their specific needs. However, we initiate the PoC with the understanding that, if successful, the customer will enter into an annual contract with us. This ensures that we are not just providing a temporary solution but establishing a long-term relationship with the customer.
Phase 3: Multi-Year Contracts
Once the pilot is completed successfully, we move into the third phase, where we transition the customer engagement to a commercial contract that includes all the requirements, models, and services the customer needs. Our goal is to move towards multi-year contracts, which provide stability and long-term partnership opportunities.
In summary, we use a phased approach to engage with customers, starting with trials, moving into pilots, and then transitioning into commercial contracts. Our pricing is based on the scope of the solution, which is determined by how many models the customer requires. This structure has helped us establish strong, long-term relationships with customers while ensuring that our revenue aligns with the value we provide.
(4) Marketing and Sales
Marketing
We focus on cracking the code for marketing in a non-conventional way because I don't always find traditional frameworks useful. Instead, my approach revolves around answering a very basic yet essential question: Which customers are willing to pay, and how much? Once we know this, we can craft a message that clearly shows how our solution fits their needs.
Our marketing strategy revolves around two primary components:
- Building a strong network of lead magnets to facilitate warm introductions
- Building our brand from a thought leadership perspective through
- Customer traction,
- Technology evidence, and
- Regulatory guidelines.
This holistic approach has helped us effectively navigate the diverse market conditions in Asia and build strong relationships with our B2B customers. By focusing on these areas, we’ve been able to drive business growth and foster trust with our stakeholders.
Referrals and Lead Magnets
One of the most effective marketing channels for us has been building a network of lead magnets. These are influential figures in the industry, such as senior advisors, thought leaders, and strategic partners. For instance, we engage with prominent institutions like IMDA and SUTD, as well as our investors like Plug and Play. These entities help us stay informed and allow us to directly reach the right people.
The focus is on nurturing relationships and generating warm introductions. Instead of cold outreach, which is least effective in B2B sales, we aim for a healthy number of intros each month. These intros help us quickly assess whether a customer needs our product and shorten our sales cycle significantly.
Thought Leadership
Building our brand has been another critical aspect of our marketing strategy. Our approach focuses on three core elements of credibility:
- Customer Traction: We highlight our traction by working with high-profile customers and showcasing case studies. For example, we worked with the US Department of Homeland Security this year, and we aim to secure more prominent clients in the future. Publicly announcing these partnerships builds credibility and trust among potential clients.
- Technology Evidence: Our R&D team plays a key role here. Everything we build is backed by science, and we publish papers that undergo peer review by AI experts. This ensures that our solutions are technically sound and provides credibility within the AI community.
- Regulatory Guidelines: We actively engage with regulatory bodies to ensure our technology complies with data privacy guidelines. For instance, we have advised PDPC Singapore on two guidelines that share how businesses can comply with PDPA (Personal Data Protection Act) Singapore using data anonymization and synthetic data. This involvement demonstrates our commitment to compliance and reassures customers that we understand and deeply respect data protection laws.

Quality Content
For Betterdata, social media has not been a major driver of new business, especially since we operate in the B2B space and engage with tier-one enterprises. Currently, all of our ongoing projects are with large enterprises, and none of them came through social media. So, at least for us, we haven’t seen significant success directly from social media channels.
However, content marketing—specifically publishing technical papers—has played a crucial role. For example, one of our projects in Hong Kong started because a potential customer read one of our technical papers published in IEEE. They reached out to us referencing the paper, which led to a swift pilot project and a growing relationship. This shows that while social media hasn’t been a major source of leads, the technical content we produce can still generate high-quality inbound interest.
We’ve recently focused more on the quality of the content we create, aiming to produce pieces that address real problems our customers face, rather than generic content. This shift has led to a noticeable increase in high-quality inbound leads. For example, one recent lead came from a senior architect at eBay in the US, who reached out after reading a blog we wrote about an overview of synthetic data.
While social media specifically hasn’t been a primary tool for lead generation, high-quality content that is well-informed by customer needs has been a valuable asset. Over time, we’ve seen a clear correlation between high-quality content and inbound interest, suggesting that content marketing—especially when it speaks to real customer challenges with depth and length—can still be an effective strategy even in a B2B context.
Sales
Using Case Studies
For sales in B2B software, the most critical factor is case studies. In Asia, especially, there's a strong focus on risk aversion, meaning potential customers often want to know who else is using the product before making any commitments. If you don't have case studies to show, you need to build credibility through other means, such as demonstrating customer traction, providing proof of your technology, and adherence to regulations. This triangle of credibility—customer traction, technology, and regulatory validation—helps customers feel more comfortable with your product and your company's reliability.
Pricing-to-Evidence Ratio
When it comes to pricing, there's a clear correlation between the size of the deal and the level of evidence you need to present. For a smaller deal, such as $10,000, you might only need some basic feedback or initial interest. But as the price increases—say, to $100,000—you'll need much more evidence to justify that cost. Potential customers will ask a lot of questions about your product’s differentiators, the frameworks you’ve used to build it, and who else is already using it.
It’s a balancing act. You can’t ask someone to pay $100,000 for a product with no evidence (unless you’re from OpenAI, pun intended), but you also can’t expect to build credibility while offering your product for free. Finding that balance requires a lot of research, customer interactions, and, often, luck. As a startup, you’ll have to be persistent, finding the believers who are willing to take a chance on you while understanding that not everyone will. Out of 100 potential customers, maybe only one will be a true believer. But it’s that one that can make all the difference.
The curve from zero to $100K is more exponential than linear. Sales and decision-making are rarely straightforward or linear; they are often non-linear and driven by both logic and emotion. A lot of times, you’ll encounter stakeholders who are unsure or even unaware of what they really need, and in those situations, even with strong evidence, you might still face obstacles.

Sometimes, you might luck into an introduction—like knowing the president's son, for example—and land a big deal, but then the challenge remains, how to deliver on that deal. This is where evidence isn't just about showing that your product works, but also about demonstrating that you can execute effectively. You need to guide the customer in the right direction, showing them not only that your product can solve their problem but also that you know how to break down the requirements, define milestones, and ultimately deliver value. The evidence you build must align with guiding the customer through their decision-making process. As their conviction grows—seeing results, understanding the process, and getting to a point where they feel confident in your ability to deliver—that’s when they’ll be ready to commit long-term.
In essence, the process involves building trust and credibility over time, leveraging case studies and evidence to justify pricing, and constantly searching for those who see the potential in your product. It’s about efficiently using your time and resources to transition from a problem-solution fit to product-market fit.
(5) Fundraising and Growth
For fundraising, we took an unconventional approach, raising funds when we didn't necessarily need to, rather than waiting until the last minute. We also put a lot of effort into creating a detailed investment memo that made it easy for investors to extract the key information.
Fundraising
In terms of advice for other founders, here are two key takeaways:
- Fundraise when you don’t need to
- Be very, very mindful of how you present information
Fundraise When You Don't Have To
We closed our seed round in April 2023, a time during which we weren’t actively raising. Founders should always have a fundraising mentality. I wouldn’t recommend waiting until you have just a few months of runway left. This is cutting it too close. Instead, stay in fundraising mode actively, and when the timing is right, execute. How do you know when the timing is right? It is when investors are reaching out to you, not the other way around.
For us, our investment came through a mix of referrals and inbound. We didn’t start fundraising in August 2022, but we kept conversations going, and eventually, things moved forward. Even our lead investor initially rejected us. They said we weren’t the right fit, but a few months later, we simply re-engaged again and things fell into place. I cannot emphasize the importance of staying humble and treating everyone you meet with humility, because you never know, they can always come back and make things work.
The key here is to always have a fundraising mindset. You don’t need to be in panic mode with only a few months of runway left. Instead, keep your pipeline open and always be building relationships and networking. This way, when the time is right, you can execute quickly.
Be Very, Very Mindful of How You Present Information
For fundraising, one of the most effective strategies we used was creating a comprehensive, easily digestible investment memo. We built this memo on Notion, which covered everything: the problem we’re solving, the solution, the market, the team, risks, challenges, and opportunities. It also included a data room with all the necessary details in one place. The goal was for any investor to review the memo and data room within 30 minutes, getting a clear overview of everything without needing to chase down additional information. This approach made it easier for investors, and we received positive feedback because we made their jobs easier by providing all the necessary details upfront, saving them time. People remember you when you make their jobs easier and people forget you when you do the opposite.
We also focused a lot of attention on our financial model, especially since investors in Asia really value numbers (and I mean really!). At the seed stage, creating a 5-year financial forecast is overkill, can be tricky and is needed by almost every investor I met, but we did put a lot of thought into our assumptions and made sure the numbers made sense. In retrospect, I still believe it is an overkill because I have yet to meet a startup in Asia who said they hit their projections, but the financial model can still be used as a proxy to understand what kind of business you want to build.
Another key aspect of our approach was the format of the data room. We ensured that all the information was well-organized and easy to navigate. Instead of bombarding investors with numerous files that would take hours to go through, we focused on making each file concise and clear, so they could quickly grasp the key details. As the old adage goes, brevity is the soul of wit and that is exactly what we did.
The Pitch
When it comes to crafting and conducting a pitch, my approach is to keep things simple. At the seed stage, I focus on four main things: problem, solution, team, and market—that's it. The key litmus test for me is how well I can summarize each of these aspects in just a few words, eliminating unnecessary details while still communicating the full picture.
The goal is to find the few words that convey the essence of the message. Being able to communicate everything effectively in under a minute is an excellent stress test. It forces you to find words that are meaningful and precise, especially when you’re pitching.
For later stages, you can expand on things like market segments, traction, acquisition rates, retention rates, and logos you’re working with. But for seed stage, focus on those four key areas. I would say you need to be particularly strong in at least three of those four areas. If you can do that, you’ll paint a compelling picture.
Growth
Clearly Define Your Goal and Strategy
When it comes to growth and scaling, I believe it’s essential to have a clear goal or sense of direction. Let me explain it like this: Imagine I’m hungry and need to find a restaurant. There are restaurants all around me—360 degrees, the full circle—but I have to choose one. Once I decide, I need to start walking toward it. Every step brings me closer.
Two critical factors here: First, choosing the right restaurant (or, in business terms, setting the right goals). Second, understanding the timeframe and the steps required to reach those goals.
Prioritise Consistency Over Effort
This is where discipline comes in. What you can’t afford to do is set a goal, take two steps, then change your mind and start walking toward another goal. If you keep jumping from one thing to the next, you’re chasing your own tail—you’re not progressing in any specific direction and that is super-extra-very unproductive. The secret to growth is consistency, not necessarily hard work. It’s the consistency of doing something over time that allows you to gain insights and understanding that others can’t easily replicate. This only happens if you focus on something for a prolonged period.
This is crucial, especially in today's world being constantly distracted by things like TikTok and YouTube, where we can skip or speed up content. We think we control everything, but when it comes to goals, that’s not how it works. Especially when things aren’t going as planned, you have to stand your ground. You can’t just change targets every time things get tough. When things get tough, the tough get going.
Double Down on Your Strengths
It's important to have agreement within your team, whether it's just one person or 100. You need to clearly define your strengths and weaknesses. Once you know what your strengths are, double down on them and create a plan to achieve your goals. Then, stick with that plan for a set time—say X months, define ownership for each team member and hold them accountable.
Ensure The Team is Aligned
The key is to commit to your decision and see it through to completion. This is what leads to real results. Many people confuse hard work with grinding away endlessly without taking time to learn and reflect. If you’re working 24/7 but not gaining insights, then I assure you, you are on the wrong path. So, it’s crucial to find your goal post and align with your team in that direction.
To give you a personal example: I’m a Muslim, and we pray five times a day. When we pray, we face the Kaaba, a place of worship in Mecca, Saudi Arabia. This direction is essential—without it, everyone would be praying in different directions, creating chaos. This very alignment, the sense of direction, is what brings unity, both in our personal and professional lives. By extension of logic, in business, alignment with your team on the goal post ensures everyone is moving in the same direction, leading to compounding and multiplying results. That is the true secret of growth.
Once you define your goals and focus on your strengths, you’ll either achieve them or you won’t. If you hit your goals, great—you can push beyond them. If not, step back, reassess, and refine your strategy. You can always adjust your approach, but the key is to keep moving toward that specific goal post.
(6) Team-Building and Leadership
Co-Founders
When it came to finding a co-founder, I relied more on intuition than a rigid framework. I was looking for someone who could complement my skills and approach problems from different angles. I met my co-founder, Kevin, during the Entrepreneur First program. At that point, we were both in teams that had fallen apart. We had six weeks to come up with something to pitch, so there was urgency. We discussed several ideas, and I liked how my co-founder approached problems from multiple perspectives. He was thinking about engineering solutions, considering both short-term inconveniences for long-term gain, and vice versa. This idea of “short-term inconvenience equals long-term convenience” resonated with me.
After a few meetings, I just had this gut feeling that he was someone I could work with. On the flip side, Kevin, came to me with a framework—a list of 100 questions to ask your potential co-founder. That’s the opposite of how I operate. I remember vividly we were sitting at a restaurant in Funan Mall, Singapore, when he presented it to me. I told him straight away that it wasn’t my style. I don’t need to answer 100 questions. I’ve made my decision that we can work together, and that’s it.
For his own peace of mind, I did however tell him that he could ask me a few important questions that mattered most to him. And so, we did. It was a moment that really highlighted the contrast in how we approach things. My approach is more non-linear, while his is more structured and methodical. It’s a great fit for us because it allows us to approach problems from different angles, and that’s been invaluable in running the company together.
Employees
When it comes to finding talent for my startup, I’ve learned that there’s no one-size-fits-all answer. The moment I think I’ve found the most important factor, something changes and my perspective shifts. But, generally, I value talent on two fronts:
- Ability to handle uncertainty, and
- Cultural fit.
First, uncertainty. I always ask candidates how they handle uncertainty and for a specific example. This can be personal or professional. The way they walk me through their process gives me insight into how they would handle the uncertainty that comes with working at a startup. In a startup, you're often figuring things out on your own, with no roadmap. You need to be independent and self-reliant, believing that no matter what, you'll figure it out. So how someone handles uncertainty is a key indicator for me in determining whether they’re a good fit to be our team members.
Second, I believe skills can be learned over time, but culture is what keeps people at a company. People don’t usually leave because of a lack of skills; they leave because of cultural misalignment. So, it’s really important for me to ensure that there’s alignment between an employee’s personal goals and inspirations and the environment we provide. I want to create a space where every team member feels appreciated for their contributions and secure in their livelihood. I don’t want anyone distracted by concerns about their salary, equipment, or benefits. Once those basic needs are met, they can focus fully on their work.
I’ve found that some people have very specific demands, and they’re not a fit for us. But others have a strong obsession with their work, a commitment to what they do, and a unique experience they bring to the table. For these people, we try to provide the environment they need to thrive.
Within that environment, I also consider how people approach work and play. I believe there’s no substitute for work—no matter what, everyone has to work. But the second question is: how do you make work enjoyable? How do you find fun in it? Some people are very conscious of their habits. For me, video games are for my mind, badminton for my body, and cooking for my creativity. But some people think they can work 24/7 without taking a break, and I always cringe at that. It’s important to have hobbies or habits that give you creative freedom and allow you to think outside the box. This is what, I believe, ultimately helps in building a successful startup.
So, zooming out, the two main things I focus on are: 1) how someone handles uncertainty, which reveals a lot about their character, and 2) whether someone fits within our work culture such that we can provide the ideal environment where they feel appreciated for their contributions and secure in their personal well-being. Once we find alignment on these two fronts, we make the offer.
Team Management and Operations
When it comes to team management and operations, I like to tell my team that I’m not the CEO (Chief Executive Officer), but rather the "Chief Easiness or Convenience Officer" if you may. My role is to make sure everything runs smoothly and take care of anything the team needs. I work to remove obstacles, so the team can focus on execution. I solve problems and problems solve me, and I enjoy it.
Internally, our team is split into two main areas: the backend (internal team) and the frontend (market-facing team). There is no hierarchy between us internally. We define our goals based on what needs to be developed, assign tasks, and then meet weekly as a company to share updates and review progress. The key here is full ownership of tasks and accountability for getting things done. I also help with product development, as I have a sense of all the challenges the company is facing, and my goal is to solve them and clear the way for others.
Backend Team
Since there is no formal hierarchy within our internal team, tasks are assigned according to scope of work defined by customers, and we all operate as team members. Our approach is similar to that of a sports team—sometimes someone excels, and other times they might struggle, but we all move together. There is no blame; we win or lose as a team. We support each other and execute together. If I'm not pulling my weight, it’s not fair to be on the team. We emphasize the importance of complementing each other and creating an environment where everyone can thrive.
As for process, we allow creative freedom in how people work, but we establish some anchors, such as defining key meetings and regular check-ins. Once tasks are clear, everyone has the freedom to work in the way that suits them best.
Frontend Team
For the market-facing side, there’s more of a hierarchy, as this reflects the expectations of the market. If I introduce myself as the "CEO," the perception is different than if I say I’m the "Chief Janitor Officer." This distinction impacts how we communicate and build our brand. We divide this front-end team into four main areas:
- Business
- Product
- Software Engineering
- Machine Learning Engineering
- R&D
We have the C-suite at the top, followed by team leads, and then the executors. The key here is that we take market expectations and distill them down into actionable tasks.
The process and workflow looks like this:
- Market expectations are received.
- Tasks are defined and assigned to the team.
- Execution happens with no communication barriers between team members. Everyone can talk to anyone, and the focus is always on getting things done effectively.
The Tech
Deep Learning, Generative AI, Synthetic Data and Privacy Engineering
The Problem: Using Valuable Data Without Breaching Privacy
Let’s start with a simple example — banks. Banks collect a lot of personal data from their customers, including transaction data (for example, purchases you make with your bank card). This data is incredibly valuable because it can be used to understand spending habits and personalize services (like recommending products or services based on your preferences). But here’s the catch: you would never want to share your personal transaction data with anyone unless you trust them completely.
Why? Because privacy is a fundamental concern. Every person’s financial data is private, and it’s protected by law (like GDPR, PDPA, etc.). For a bank with millions of customers, this becomes a huge challenge. The bank wants to use the data for innovation — such as offering personalized promotions or improving services — but it cannot because the data is private. So, what can they do?
The Solution: AI and Synthetic Data Generation
That’s where our platform comes in. The solution we’ve built uses AI (specifically generative models and privacy-enhancing technologies) to tackle this privacy challenge. Here's how it works:
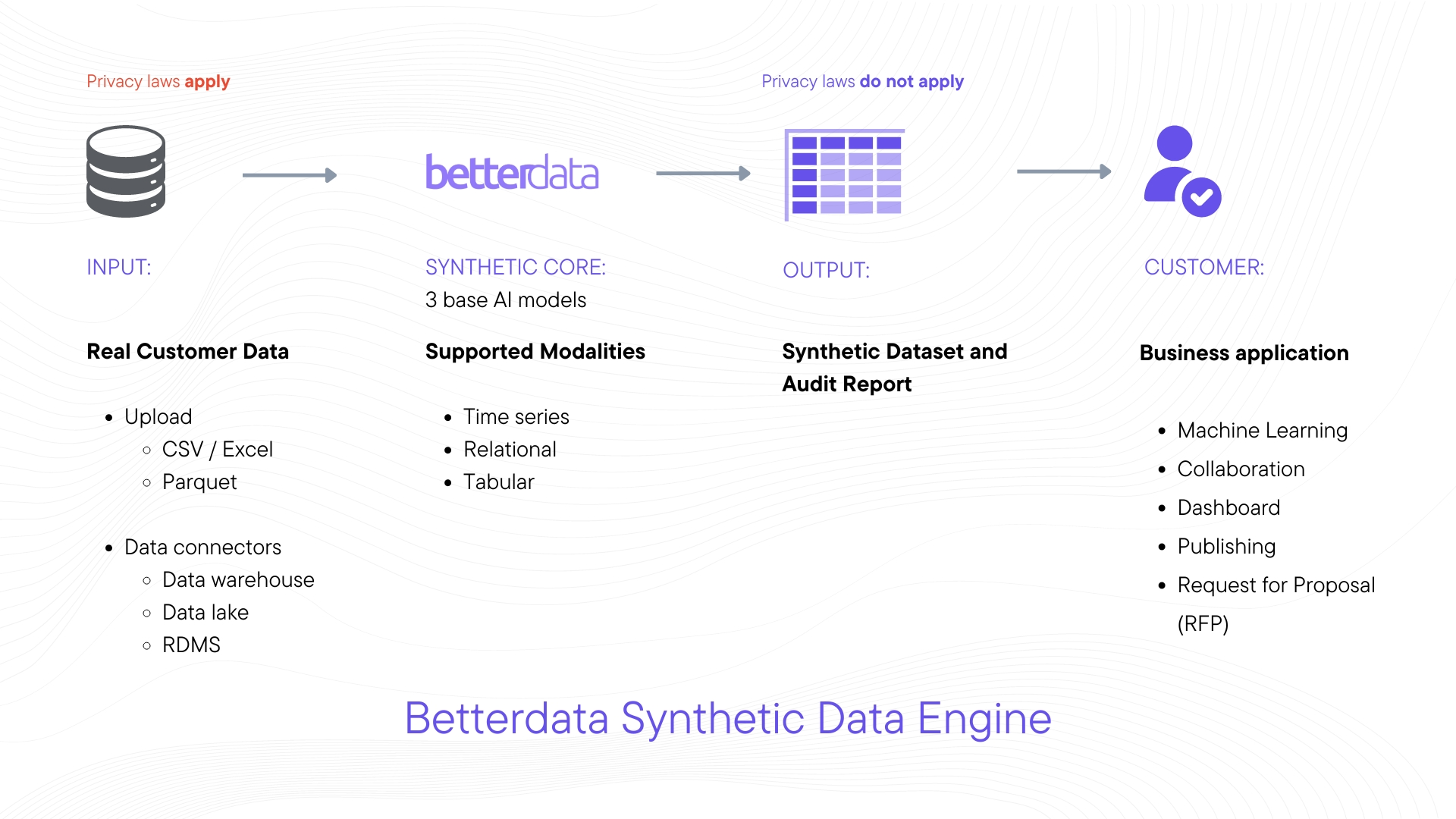
Step 1: AI Model Training
The bank has access to real customer data (but they don’t need to send this data to us). Instead, they install our platform on their systems. The bank inputs its real data into our platform, which is where the AI magic begins. The AI models are trained on this real customer data.
Step 2: Understanding the Structure of Data
The AI doesn’t directly understand or store sensitive data like transaction details, but it learns the structure of the data. For instance, it learns things like:
- What types of products or services are customers purchasing
- What time of day do customers typically make purchases
- What demographic group tends to spend more on certain items
This happens quickly (sometimes in a few hours, or maybe a few days depending on the data size and compute available), and the AI learns the "patterns" in the data.
Step 3: Generating Synthetic Data
After this training, the platform can generate new, artificial data that has the same structure and qualities as the original data, but doesn’t contain any actual private information. This data looks and behaves similarly to the real customer data but doesn’t belong to any individual customer. It’s now safe to use.
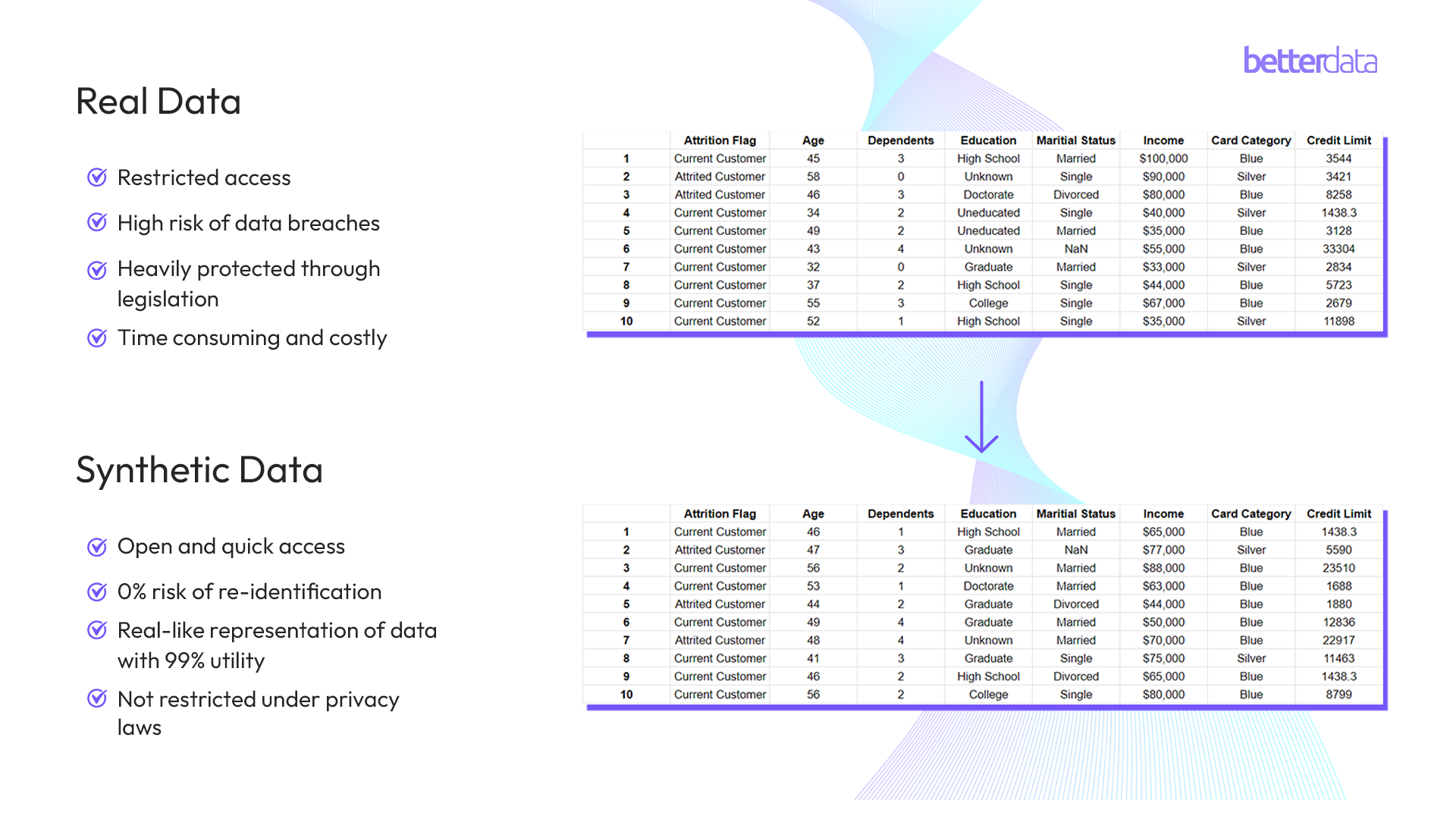
Step 4: Using the Synthetic Data
Since this data is artificial, the bank no longer needs to ask for customer consent to use it. The data isn't real, so it doesn't violate any privacy laws. The bank can now use this synthetic data for innovation — like developing new financial products, personalizing services, or conducting research without ever exposing real customer information.
The Challenge of Data Scarcity
Initially, we built this technology to solve privacy issues, especially for sectors like banking. But over time, we realized that synthetic data can also address a much broader problem: the scarcity of high-quality data for training AI models. As companies release more Large Language Models (LLMs) and other AI systems, there’s a growing need for more diverse, high-quality data to improve these systems.
In the next few years, the world will run out of real-world data to train all AI models. We could hit a plateau where there simply isn’t enough new, useful data available. But synthetic data can step in to solve this problem. By simulating data that doesn't exist in real life, we can generate new scenarios and edge cases to help AI models get smarter. For example, instead of just having data about people working 9-to-5 jobs, we could simulate data for scenarios like people working night shifts or traveling for work.
This type of simulation is crucial because AI needs variety in data to make better predictions, generate accurate insights, and perform tasks effectively. Synthetic data provides that flexibility — it can simulate anything we need, even things that might never happen in the real world.
The Bigger Picture: Privacy + Simulation = Better AI
At the core of our platform is the belief that AI must be built on the foundations of privacy. By default, privacy has to be the foundation of everything we do with AI. If you start with private data and ensure it's handled ethically, then you can create much better AI systems that are trustworthy and fair. Ultimately, our goal is to help accelerate AI innovation in all industries, which we do by providing companies with synthetic data that doesn't breach privacy regulations and personal data rights.
Here’s our technological development journey so far:
- In 2021, we started with data privacy as our main focus. We wanted to help companies use customer data without violating privacy laws.
- Now, we’ve expanded to use synthetic data to help companies train AI models and build better solutions.
- Our long-term goal is to be able to simulate any type of data we need to continue improving AI — whether it's for finance, healthcare, government, or any other industry.
The Future of AI with Betterdata
In a nutshell, our platform solves two major challenges:
- Data Privacy: By generating synthetic data that mirrors real-world data, we protect individuals' privacy while still allowing businesses to innovate.
- Data Augmentation: As the demand for AI grows, synthetic data enables companies to simulate and create new data scenarios that aren’t available in the real world, helping AI models to improve.
With this approach, we’re not just creating a tool — we’re paving the way for a future where AI can innovate ethically and businesses can harness data without compromising privacy.
Key Takeaways
- Synthetic Data is artificial data that mimics real-world data but doesn’t contain any personal or sensitive information.
- Generative AI models are trained on real data to learn patterns, and then use those patterns to create synthetic data that can be used safely.
This approach solves 2 key challenges: privacy protection and data scarcity for AI training.
Member discussion